AI-Powered Search Engines: Revolutionizing SEO
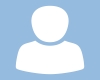
Posted by tahir
from the Search Engine Optimization category at
27 Dec 2024 05:25:40 pm.
Introduction
The advent of artificial intelligence (AI) is reshaping various industries, and search engine optimization (SEO) is no exception. Traditional search engines relied heavily on keyword-based algorithms, which often led to a narrow and sometimes inaccurate representation of what users were seeking. However, the introduction of AI-powered search engines marks a significant transformation in how we access and interact with information online.
AI-powered search engines utilize sophisticated technologies such as machine learning, natural language processing (NLP), and deep learning to understand and interpret user queries more effectively. This evolution means search engines can now comprehend the context and intent behind searches, rather than just matching keywords. As a result, users receive more relevant and accurate search results, enhancing their overall search experience.
For SEO practitioners, this shift necessitates a reevaluation of strategies. No longer can businesses rely solely on keyword density and backlinks to improve their search rankings. Instead, there is a growing emphasis on creating high-quality, engaging content that genuinely meets the needs and interests of users. Furthermore, AI-driven search engines prioritize user experience, meaning factors like page load speed, mobile-friendliness, and user engagement are more crucial than ever.
In essence, AI-powered search engines are not just enhancing the efficiency and accuracy of searches; they are also setting new standards for SEO practices. This revolution compels businesses to adopt a more holistic approach to SEO, focusing on delivering genuine value to users and fostering a more intuitive and satisfying online search experience.
Overview of SEO: Brief history and purpose of SEO.
Early Days of Search Engines
Search engine optimization (SEO) has evolved alongside the development of the internet and search engines. In the mid-1990s, the internet was expanding rapidly, and early search engines like Lycos, AltaVista, and Yahoo! were created to help users find relevant information. These search engines relied on basic algorithms that indexed web pages based on the presence of keywords and simple metadata. During this period, SEO was rudimentary, focusing primarily on keyword placement and meta tags.
The Rise of Google
The landscape of SEO changed dramatically with the introduction of Google in 1998. Google's PageRank algorithm revolutionized search by prioritizing web pages based on the number and quality of links pointing to them. This shift emphasized the importance of backlinks, signaling a more sophisticated understanding of a page's relevance and authority. SEO strategies evolved to include link-building efforts, as websites sought to improve their rankings by gaining more high-quality inbound links.
Algorithm Updates and Penalties
As Google's search algorithm became more advanced, it introduced several updates to combat spammy and manipulative SEO practices. Notable updates include:
Google Panda (2011): Targeted low-quality content and content farms, rewarding websites with high-quality, original content.
Google Penguin (2012): Focused on penalizing sites engaged in black-hat SEO techniques like keyword stuffing and unnatural link building.
Google Hummingbird (2013): Improved understanding of natural language and search query intent, enhancing the relevance of search results.
These updates underscored the need for ethical SEO practices and the creation of valuable content that genuinely addressed user needs.
The Mobile Revolution
The proliferation of smartphones brought another significant change to SEO. With more users accessing the internet via mobile devices, Google introduced mobile-friendly algorithms and emphasized the importance of responsive web design. In 2015, Google released the Mobilegeddon update, which prioritized mobile-friendly websites in search results. This shift forced businesses to optimize their websites for mobile users, further emphasizing the user experience in SEO strategies.
The Role of AI and Machine Learning
In recent years, AI and machine learning have become integral to SEO. Google's RankBrain, introduced in 2015, utilizes machine learning to better understand search queries and provide more relevant results. AI-driven search engines focus on understanding user intent, context, and behavior, moving beyond simple keyword matching. This evolution has pushed SEO strategies towards creating high-quality, user-centric content that answers users' questions comprehensively.
Purpose of SEO
The primary purpose of SEO is to improve a website's visibility in search engine results, thereby attracting more organic (non-paid) traffic. Effective SEO enhances a website's relevance and authority in the eyes of search engines, leading to higher rankings. Key objectives of SEO include:
Increasing Organic Traffic: Driving more visitors to a website through improved search engine rankings.
Enhancing User Experience: Creating a seamless, enjoyable experience for users, which indirectly boosts search rankings.
Building Brand Credibility and Authority: Establishing a website as a trusted source of information through high-quality content and ethical SEO practices.
Boosting Conversion Rates: Turning website visitors into customers by providing valuable content and a user-friendly experience.
SEO has come a long way from its early days of keyword stuffing and basic link building. Today's SEO requires a comprehensive approach that focuses on user intent, high-quality content, and ethical practices. As search engines continue to evolve with advancements in AI and machine learning, the importance of delivering genuine value to users becomes ever more paramount.
Introduction to AI-Powered Search: Definition and significance of AI in search engines.
Definition of AI-Powered Search
AI-powered search refers to the use of artificial intelligence (AI) technologies to enhance the functionality and accuracy of search engines. Unlike traditional search algorithms that rely primarily on predefined rules and keyword matching, AI-powered search engines employ advanced techniques such as machine learning, natural language processing (NLP), and deep learning to understand and interpret user queries. These technologies enable search engines to deliver more relevant and personalized search results by comprehending the context, intent, and nuances behind search queries.
Components of AI-Powered Search
Machine Learning: Machine learning algorithms enable search engines to learn and improve from data without explicit programming. By analyzing user behavior, search patterns, and feedback, these algorithms refine their understanding of what constitutes relevant search results, enhancing their accuracy over time.
Natural Language Processing (NLP): NLP allows search engines to process and understand human language in a way that is more natural and intuitive. This includes grasping the meaning behind words, recognizing synonyms, and understanding the context of phrases. As a result, search engines can better interpret user queries and deliver results that align with the user's intent.
Deep Learning: Deep learning, a subset of machine learning, uses neural networks to model complex patterns and relationships in data. In the context of search engines, deep learning helps in understanding and predicting user intent, personalizing search results, and improving the overall search experience.
Significance of AI in Search Engines
AI-powered search engines represent a significant leap forward in how we access and interact with information online. The integration of AI into search engines brings several key benefits:
Improved Search Accuracy: Traditional search engines often relied on keyword frequency and backlinks to determine the relevance of search results. AI-powered search engines, however, can analyze the context and intent behind user queries, leading to more accurate and relevant search results. This reduces the likelihood of users encountering irrelevant or low-quality content.
Enhanced User Experience: By understanding the nuances of user queries and delivering personalized results, AI-powered search engines provide a more intuitive and satisfying search experience. Users can find the information they need more quickly and efficiently, improving overall satisfaction.
Better Content Understanding: AI technologies like NLP and deep learning enable search engines to better understand and interpret web content. This means that search engines can recognize high-quality, informative content and rank it higher in search results, promoting the creation of valuable content.
Voice Search Optimization: With the rise of voice-activated assistants like Siri, Alexa, and Google Assistant, voice search has become increasingly popular. AI-powered search engines are adept at processing and understanding voice queries, which often differ from text-based searches. This capability enhances the accuracy and relevance of voice search results.
Continuous Learning and Adaptation: AI-powered search engines are designed to learn and adapt over time. By continuously analyzing user behavior and feedback, these systems can refine their algorithms and improve their performance. This ongoing learning process ensures that search engines remain effective and relevant in the face of evolving user needs and search trends.
Combating Spam and Manipulative Practices: AI-powered search engines are better equipped to detect and penalize manipulative SEO practices such as keyword stuffing, cloaking, and link schemes. By prioritizing high-quality, user-centric content, these search engines help maintain the integrity of search results and promote ethical SEO practices.
In summary, AI-powered search engines are transforming the landscape of search and SEO. By leveraging advanced technologies like machine learning, natural language processing, and deep learning, these search engines deliver more accurate, relevant, and personalized search results. This revolution not only enhances the user experience but also encourages the creation of high-quality content and ethical SEO practices. As AI continues to evolve, its impact on search engines and SEO will only grow, shaping the future of how we access and interact with information online.
Evolution of Search Engines
The evolution of search engines has been a remarkable journey, transforming the way we access and interact with information on the internet. From the humble beginnings of basic keyword searches to the sophisticated AI-powered systems we use today, search engines have undergone significant changes to enhance accuracy, relevance, and user experience.
Early Search Engines
The origins of search engines can be traced back to the early 1990s when the internet was gaining popularity. The first search engines, such as Archie, Veronica, and Jughead, were simple tools designed to index and search the contents of FTP sites. These early systems were limited in scope and functionality, primarily focused on file retrieval rather than web page content.
The Rise of Web Search
As the World Wide Web expanded, there was a growing need for more advanced search capabilities. In 1993, the World Wide Web Wanderer was developed, marking one of the first efforts to index web pages. This was followed by the launch of the first web search engine, World Wide Web Worm (WWWW), which indexed both web pages and links.
In 1994, WebCrawler was introduced as the first search engine to index the full text of web pages. This innovation allowed users to search for specific words within web pages, significantly improving search accuracy. Around the same time, Lycos, another early search engine, began indexing web pages and incorporating a ranking system based on the frequency of keywords.
The Emergence of Google
The search engine landscape was forever changed with the introduction of Google in 1998. Founded by Larry Page and Sergey Brin, Google introduced the PageRank algorithm, which ranked web pages based on the number and quality of links pointing to them. This approach provided more relevant and reliable search results, quickly establishing Google as the dominant search engine.
Google's success can be attributed to its continuous innovation and commitment to improving user experience. Over the years, Google introduced numerous features and updates, such as Google Images, Google News, and Google Maps, expanding its functionality beyond simple web searches.
Algorithm Updates and Quality Improvements
To maintain the quality and relevance of search results, Google and other search engines implemented various algorithm updates. Some notable updates include:
Google Panda (2011): Targeted low-quality content and content farms, promoting high-quality, original content.
Google Penguin (2012): Focused on penalizing websites engaged in manipulative SEO practices like keyword stuffing and unnatural link building.
Google Hummingbird (2013): Improved understanding of natural language queries and user intent, enhancing search relevance.
These updates underscored the importance of ethical SEO practices and the creation of valuable content that genuinely addressed user needs.
The Mobile Revolution
The proliferation of smartphones and mobile internet access brought significant changes to search engines. With more users accessing the internet via mobile devices, search engines had to adapt to the unique needs and behaviors of mobile users. Google introduced the Mobilegeddon update in 2015, prioritizing mobile-friendly websites in search results. This shift emphasized the importance of responsive web design and optimizing websites for mobile users.
Voice Search and AI Integration
In recent years, the integration of artificial intelligence (AI) and machine learning has revolutionized search engines. Technologies like natural language processing (NLP) and deep learning enable search engines to understand and interpret user queries more effectively. AI-powered search engines, such as Google's RankBrain, focus on understanding user intent and context, delivering more accurate and personalized search results.
The rise of voice-activated assistants like Siri, Alexa, and Google Assistant has also transformed search behavior. Voice search queries tend to be more conversational and natural, requiring search engines to process and understand spoken language. This shift has further highlighted the importance of AI and NLP in modern search engines.
The Future of Search Engines
The evolution of search engines is far from over. As AI and machine learning technologies continue to advance, search engines will become even more sophisticated in understanding and predicting user needs. The future of search may involve more personalized and context-aware experiences, where search engines anticipate user queries and provide information proactively.
Additionally, the integration of augmented reality (AR) and virtual reality (VR) could transform how we search for and interact with information. Imagine using AR glasses to visually search for products or using VR to explore virtual environments and gather information.
The evolution of search engines has been a journey of continuous innovation and adaptation. From the early days of basic keyword searches to the advanced AI-powered systems of today, search engines have transformed the way we access and interact with information. As technology continues to evolve, search engines will undoubtedly play an even more integral role in our digital lives, providing increasingly accurate, relevant, and personalized experiences.
Traditional Search Engines: How traditional algorithms worked.
Traditional search engines laid the groundwork for the advanced AI-driven systems we use today. These early algorithms focused on keyword matching and basic metrics to rank web pages. Here’s a closer look at how they worked and their foundational principles.
Keyword Matching
At the core of traditional search engines was keyword matching. When a user entered a query, the search engine would break it down into individual keywords. It then searched its index for web pages that contained those keywords. The more frequently the keywords appeared on a page, the higher the page was ranked in the search results. This method, though simple, often led to results that were not fully relevant to the user’s intent.
Metadata and HTML Tags
To improve search accuracy, traditional algorithms also relied heavily on metadata and HTML tags. Webmasters could include meta tags in the HTML code of their pages, providing information about the content. Important meta tags included the title tag, description tag, and keyword tag. The title tag indicated the page’s main topic, the description tag provided a brief summary, and the keyword tag listed relevant keywords. Search engines used this metadata to better understand the content and relevance of web pages.
Backlinks and PageRank
One of the significant advancements in traditional search algorithms was the introduction of backlinks as a ranking factor. Google’s PageRank algorithm, developed by Larry Page and Sergey Brin, revolutionized the search landscape by using the quantity and quality of links pointing to a page to determine its importance and relevance. Each link acted as a vote of confidence, with links from authoritative sites carrying more weight. This method helped prioritize high-quality content and reduced the influence of keyword manipulation.
Content Relevance and Freshness
Traditional search engines also considered content relevance and freshness. Relevance was determined by how well the content on a page matched the keywords in the user’s query. Freshness referred to the recency of the content. Search engines favored newer content, especially for queries related to current events or rapidly changing topics. This encouraged webmasters to keep their content updated.
On-Page SEO
On-page SEO techniques were crucial for traditional search engines. This included optimizing the use of keywords within the content, ensuring the site’s structure was crawlable, and improving user experience. Elements like header tags (H1, H2, H3), internal linking, and image alt text played a role in how search engines understood and ranked pages.
Crawling and Indexing
The process of crawling and indexing was fundamental to how traditional search engines worked. Crawlers, also known as spiders or bots, scanned the internet for new and updated content. These crawlers followed links from one page to another, collecting data and adding it to the search engine’s index. The index was a vast database of all the pages the search engine knew about. When a user submitted a query, the search engine retrieved relevant pages from this index.
Algorithmic Updates
As the internet evolved, so did traditional search algorithms. Search engines frequently updated their algorithms to improve accuracy and combat spammy practices. Updates targeted issues like keyword stuffing, cloaking, and link farming. These changes aimed to ensure that high-quality, relevant content was prioritized in search results.
Limitations of Traditional Algorithms
While traditional search algorithms were effective to a degree, they had limitations. They struggled with understanding the context and intent behind queries. Keyword matching often led to irrelevant results, and manipulative SEO practices could game the system. The focus on keywords and backlinks sometimes overshadowed the actual quality and usefulness of content.
Traditional search engines played a critical role in shaping the internet as we know it today. By relying on keyword matching, metadata, backlinks, and on-page SEO, they provided a foundation for web search. However, their limitations highlighted the need for more sophisticated approaches, paving the way for the AI-powered search engines that have transformed how we access information in the digital age.
Introduction of AI in Search: Transition from traditional algorithms to AI-driven systems.
The integration of artificial intelligence (AI) in search engines marks a pivotal shift from traditional algorithms to more advanced, intelligent systems. This transformation has redefined how we access and interact with information on the internet, enhancing the relevance, accuracy, and personalization of search results.
Traditional Search Algorithms
Traditional search engines relied on keyword matching and basic metrics to rank web pages. These algorithms primarily focused on identifying the frequency and placement of keywords within a web page's content and metadata. Additionally, they utilized backlinks as a measure of a page's authority and relevance. While effective to a degree, these methods had limitations. They often failed to understand the context and intent behind user queries, leading to irrelevant or suboptimal search results. Moreover, they were susceptible to manipulative SEO practices such as keyword stuffing and link schemes.
The Advent of AI in Search
The introduction of AI in search engines was driven by the need to address these limitations. AI technologies, particularly machine learning, natural language processing (NLP), and deep learning, brought a new level of sophistication to search algorithms. Unlike traditional algorithms, AI-driven systems can learn from data, understand context, and adapt to user behavior over time.
Machine Learning
Machine learning is a core component of AI-powered search engines. It enables search engines to improve their performance by analyzing vast amounts of data. Through machine learning, search engines can identify patterns in user behavior, understand search intent, and refine their algorithms to deliver more relevant results. This continuous learning process allows AI-driven systems to stay current with evolving search trends and user preferences.
Natural Language Processing (NLP)
NLP is another critical technology in the transition to AI-driven search. Traditional search engines struggled with understanding natural language queries, often failing to grasp the meaning and context behind words. NLP enables search engines to process and interpret human language more accurately. It allows them to recognize synonyms, understand the nuances of phrases, and decode the intent behind queries. As a result, search engines can provide more accurate and contextually relevant results.
Deep Learning
Deep learning, a subset of machine learning, uses neural networks to model complex patterns and relationships in data. In search engines, deep learning enhances the ability to understand user intent and predict the most relevant results. It also powers features like image and voice search, enabling search engines to interpret and respond to multimedia queries effectively.
Personalization and Context Awareness
AI-driven search engines excel in personalization and context awareness. By analyzing user behavior, search history, and preferences, they can tailor search results to individual users. This personalization improves user experience, making searches more efficient and satisfying. Additionally, AI can consider contextual factors such as location, time, and device type, further enhancing the relevance of search results.
Combating Manipulative Practices
AI's ability to understand and analyze data at a deeper level helps in combating manipulative SEO practices. AI-driven search engines can detect and penalize tactics like keyword stuffing, cloaking, and link schemes, ensuring that high-quality, user-centric content is prioritized. This focus on quality content encourages ethical SEO practices and improves the overall integrity of search results.
The Future of AI in Search
The integration of AI in search engines is an ongoing process with significant potential for future advancements. As AI technologies continue to evolve, search engines will become even more adept at understanding user needs and providing proactive, context-aware information. Emerging technologies like augmented reality (AR) and virtual reality (VR) may also be integrated with AI-driven search, offering new ways to interact with and retrieve information.
The transition from traditional algorithms to AI-driven systems has revolutionized the world of search. AI-powered search engines, with their ability to learn, adapt, and understand human language, offer more accurate, relevant, and personalized search experiences. This evolution not only enhances user satisfaction but also promotes the creation of high-quality content and ethical SEO practices. As AI continues to advance, it will undoubtedly shape the future of how we search for and interact with information online.
Components of AI-Powered Search
Machine Learning: How search engines use machine learning to improve search results.
Machine learning (ML) has become a cornerstone of modern search engines, transforming the way they understand and respond to user queries. By leveraging vast amounts of data and sophisticated algorithms, search engines use machine learning to deliver more accurate, relevant, and personalized search results. Here’s an in-depth look at how machine learning improves search engines:
Understanding User Intent
One of the primary challenges for traditional search engines was accurately interpreting user intent. Machine learning addresses this by analyzing patterns in user behavior and search history. By studying how users interact with search results—such as which links they click on, how long they stay on a page, and what queries they refine—machine learning models can infer what users are truly looking for. This helps search engines provide results that better match the user's intent, even if the query is ambiguous or imprecise.
Improving Relevance
Machine learning enhances the relevance of search results by continuously learning from new data. Algorithms such as supervised learning, unsupervised learning, and reinforcement learning enable search engines to identify and rank the most pertinent information. For example, supervised learning algorithms are trained on labeled data, learning to predict the relevance of search results based on features such as keyword frequency, link quality, and user engagement metrics. Over time, these models become more adept at recognizing relevant content, leading to improved search accuracy.
Personalization
Personalization is a significant advantage of machine learning in search engines. By analyzing user data, such as search history, location, and preferences, machine learning models can tailor search results to individual users. This ensures that the content presented is not only relevant to the query but also aligned with the user’s interests and previous behaviors. Personalized search results enhance user satisfaction by delivering a more customized and intuitive search experience.
Natural Language Processing (NLP)
Natural language processing (NLP) is a subset of machine learning that focuses on understanding and interpreting human language. NLP enables search engines to process queries more naturally, recognizing nuances such as synonyms, slang, and context. This allows search engines to move beyond simple keyword matching and understand the deeper meaning behind user queries. As a result, NLP-powered search engines can provide more precise and contextually relevant results, improving the overall search experience.
Handling Voice and Image Searches
With the rise of voice-activated assistants and image search capabilities, machine learning has become crucial in processing non-text queries. Speech recognition models, powered by machine learning, can accurately transcribe spoken queries, enabling effective voice search. Similarly, image recognition algorithms allow search engines to analyze and interpret visual content, providing relevant results based on images rather than text. These advancements expand the scope and versatility of search engines, catering to a broader range of user needs.
Combating Spam and Low-Quality Content
Machine learning algorithms are adept at detecting and combating spam and low-quality content. By analyzing patterns and characteristics of known spammy or manipulative practices, such as keyword stuffing and link schemes, machine learning models can identify and penalize these behaviors. This ensures that high-quality, trustworthy content is prioritized in search results, maintaining the integrity and usefulness of search engines.
Continuous Improvement and Adaptation
One of the key strengths of machine learning is its ability to continuously learn and adapt. Search engines regularly update their machine learning models with new data, allowing them to stay current with evolving search trends and user behaviors. This ongoing learning process ensures that search engines remain effective and relevant, consistently improving their performance over time.
Machine learning has revolutionized search engines by enhancing their ability to understand user intent, improve relevance, and deliver personalized results. Through techniques like natural language processing, voice and image search capabilities, and spam detection, machine learning models ensure that search engines provide accurate, relevant, and high-quality content. As machine learning technology continues to advance, search engines will become even more sophisticated, further transforming the way we access and interact with information online.
Natural Language Processing (NLP): Understanding user queries with NLP.
Natural Language Processing (NLP) is a subset of artificial intelligence that enables machines to understand, interpret, and generate human language in a way that is both meaningful and useful. In the context of search engines, NLP plays a critical role in enhancing the accuracy and relevance of search results by comprehending the nuances of user queries.
The Basics of NLP
NLP encompasses a range of techniques and methodologies for processing natural language, including syntax analysis, semantics, discourse integration, and pragmatic analysis. These techniques allow search engines to move beyond simple keyword matching and understand the context, intent, and intricacies of user queries.
Syntax Analysis: Also known as parsing, this involves analyzing the grammatical structure of sentences. It helps in identifying parts of speech, such as nouns, verbs, and adjectives, and understanding their relationships within a query.
Semantics: This involves understanding the meaning behind words and sentences. Semantics allows search engines to recognize synonyms and related terms, ensuring that queries return relevant results even if the exact keywords aren’t used.
Discourse Integration: This technique considers the context of a query within a larger conversation or sequence of searches. By integrating previous queries and interactions, search engines can provide more coherent and contextually appropriate results.
Pragmatic Analysis: This focuses on understanding the practical aspects of user language, such as intent, tone, and implied meanings. Pragmatics help search engines infer what users are truly seeking, even if their queries are vague or ambiguous.
Enhancing Query Understanding
NLP improves search engines’ ability to interpret complex and conversational queries. Traditional keyword-based approaches often struggled with queries that included natural language elements, such as “best place to eat nearby” or “how to fix a leaky faucet.” NLP techniques enable search engines to understand such queries by breaking down the components and identifying the user’s intent.
For example, with a query like “What’s the weather like in Tokyo this weekend?”, NLP helps the search engine recognize that the user is asking for weather information for a specific location and time frame. It processes the query to deliver precise and relevant results, such as a weather forecast for Tokyo for the upcoming weekend.
Personalization and Context
NLP also plays a significant role in personalization and contextual search. By analyzing user behavior, preferences, and search history, NLP allows search engines to tailor results to individual users. This ensures that users receive information that is not only relevant to their query but also aligned with their interests and past interactions.
Additionally, NLP enables search engines to understand and incorporate contextual clues, such as location and time, to refine search results. For instance, a query for “coffee shops” might yield different results depending on whether the user is searching from New York or Tokyo.
Natural Language Processing is revolutionizing the way search engines understand and respond to user queries. By leveraging advanced techniques to analyze and interpret human language, NLP allows search engines to deliver more accurate, relevant, and personalized search results. This not only enhances the user experience but also sets new standards for search engine optimization and content creation. As NLP technology continues to evolve, its impact on search engines will only grow, making it an essential component of modern search algorithms.
Deep Learning: Role of neural networks in refining search accuracy.
Deep learning, a subset of machine learning, leverages neural networks to analyze and interpret vast amounts of data, thereby refining the accuracy of search results. Neural networks, inspired by the structure and function of the human brain, consist of interconnected nodes (neurons) organized into layers. Each layer processes information and passes it on to the next, allowing the system to learn and make complex predictions.
Understanding Neural Networks
Neural networks are composed of three main types of layers: input layers, hidden layers, and output layers. The input layer receives raw data, which is then processed by one or more hidden layers. These hidden layers perform intricate calculations and transformations, extracting features and identifying patterns within the data. Finally, the output layer produces the final prediction or result. This layered approach enables neural networks to handle high-dimensional data and uncover intricate relationships.
Enhancing Search Accuracy
Deep learning enhances search accuracy in several key ways:
Feature Extraction: Traditional search algorithms often struggle with identifying complex features within data. Neural networks, however, excel at feature extraction. Through multiple layers of processing, they can identify intricate patterns and relationships, such as understanding the context of a query or the relevance of web content. This capability allows search engines to deliver more precise and relevant results.
Contextual Understanding: Deep learning models, particularly recurrent neural networks (RNNs) and transformers, are adept at understanding context. These models can process sequences of data, such as sentences or entire documents, and maintain an understanding of context throughout. This helps search engines interpret the nuanced meaning behind user queries, even when they involve natural language or complex phrases.
Personalization: Neural networks can analyze user behavior and preferences to deliver personalized search results. By examining data such as search history, click patterns, and past interactions, deep learning models can predict what information a user is likely seeking. This personalization enhances user satisfaction by providing results tailored to individual needs and preferences.
Handling Diverse Data Types: Deep learning models are capable of processing diverse data types, including text, images, and audio. For example, convolutional neural networks (CNNs) are particularly effective at image recognition, enabling search engines to understand and respond to visual queries. This versatility expands the functionality of search engines, allowing them to cater to a broader range of user queries.
Continuous Learning and Adaptation: Deep learning models improve over time through continuous learning. As they process more data and receive feedback, they refine their understanding and predictions. This adaptability ensures that search engines remain effective in the face of evolving user behaviors and search trends.
Deep learning, with its powerful neural networks, plays a crucial role in refining search accuracy. By enhancing feature extraction, contextual understanding, personalization, and the ability to handle diverse data types, deep learning models enable search engines to deliver more accurate, relevant, and personalized results. This transformation not only improves the user experience but also sets new standards for search engine optimization and content creation. As deep learning technology continues to advance, its impact on search engines will only grow, further shaping how we access and interact with information online.
Impact on SEO Strategies
Keyword Research: Changes in keyword research and optimization.
Keyword research has always been a cornerstone of SEO, but the methods and strategies have evolved significantly with the advent of AI and changes in search engine algorithms. Here's how keyword research and optimization have changed over time:
Traditional Keyword Research
In the past, keyword research primarily involved identifying high-traffic keywords and integrating them into web content as frequently as possible. Tools like Google's Keyword Planner and other keyword research software provided lists of popular search terms, which were then used to optimize meta tags, headings, and body content. The goal was to rank highly for specific keywords by achieving a high keyword density.
Shift to User Intent
Modern keyword research has shifted from focusing solely on high-traffic keywords to understanding user intent. Search engines, powered by AI, now prioritize delivering results that best match the intent behind a query. This means that keywords must be contextually relevant and aligned with what users are actually seeking. SEO practitioners now use tools that provide insights into user intent, search trends, and long-tail keywords—phrases that are more specific and typically less competitive.
Semantic Search and NLP
With the integration of natural language processing (NLP) and semantic search, search engines can understand the meaning and context behind queries. This has led to a greater emphasis on topic clusters and related keywords rather than exact match keywords. Content is now optimized to cover broader topics comprehensively, addressing various aspects of a subject to meet different user intents.
Voice Search and Conversational Queries
The rise of voice search has further transformed keyword research. Voice queries tend to be more conversational and longer than text queries. As a result, SEO strategies now include optimizing for natural language phrases and questions that users might ask their voice-activated assistants.
In summary, keyword research and optimization have evolved from simple keyword density tactics to sophisticated strategies focused on user intent, context, and natural language. By understanding these changes and leveraging advanced tools, SEO practitioners can create more relevant and engaging content that meets the needs of modern search engines and users.
Content Creation: Importance of quality content over keyword stuffing.
In the early days of SEO, keyword stuffing—cramming as many keywords as possible into web content—was a common tactic used to manipulate search engine rankings. While this strategy might have temporarily boosted rankings, it often resulted in poor-quality content that was difficult to read and provided little value to users. As search engine algorithms have evolved, the importance of quality content over keyword stuffing has become paramount.
User Experience and Engagement
Modern search engines, powered by AI and natural language processing, prioritize user experience and engagement. Quality content that is informative, engaging, and easy to read is more likely to satisfy user intent, leading to longer page visits, lower bounce rates, and higher user satisfaction. These factors signal to search engines that the content is valuable, thereby improving its search ranking.
Relevance and Context
Search engines now understand the context and relevance of content better than ever before. Quality content provides comprehensive coverage of a topic, addressing various aspects and answering potential questions users might have. Instead of focusing solely on keyword frequency, creating content that is thorough and well-researched helps build authority and trust, which are crucial for higher search rankings.
SEO Best Practices
Current SEO best practices emphasize the creation of high-quality content that naturally incorporates relevant keywords. This includes using keywords in a way that flows naturally within the text, ensuring that the content is meaningful and valuable to the reader. Quality content also includes elements like images, videos, and infographics, which enhance understanding and engagement.
Avoiding Penalties
Keyword stuffing is not only ineffective but can also lead to penalties from search engines like Google. Algorithms such as Google Panda and Penguin specifically target and penalize websites that use manipulative practices. Maintaining a focus on quality content helps avoid such penalties and ensures that the website adheres to ethical SEO guidelines.
In summary, the importance of quality content over keyword stuffing cannot be overstated. By prioritizing user experience, relevance, and ethical SEO practices, content creators can improve their search rankings and provide genuine value to their audience. This approach not only enhances the effectiveness of SEO strategies but also fosters long-term success in the digital landscape.
User Experience: Focus on user satisfaction and engagement.
In the realm of SEO and web design, user experience (UX) has emerged as a critical factor in determining the success of a website. Modern search engines, driven by advanced AI algorithms, prioritize websites that deliver a superior user experience, making user satisfaction and engagement paramount for achieving high search rankings.
Importance of User Satisfaction
User satisfaction is the cornerstone of effective UX design. When users find a website easy to navigate, informative, and engaging, they are more likely to stay longer, interact with the content, and return in the future. Positive user experiences lead to lower bounce rates, higher time-on-page metrics, and increased page views—all of which signal to search engines that the content is valuable and relevant.
Key Elements of User Engagement
Several elements contribute to user engagement and satisfaction:
Intuitive Navigation: A well-organized website with clear, logical navigation helps users find what they need quickly and efficiently. This reduces frustration and enhances the overall user experience.
Responsive Design: With the proliferation of mobile devices, having a responsive design that adapts to different screen sizes is crucial. Mobile-friendly websites ensure that users have a seamless experience, regardless of the device they use.
Page Load Speed: Slow-loading pages frustrate users and lead to higher bounce rates. Optimizing page load speed by compressing images, leveraging browser caching, and minimizing code enhances user satisfaction.
Quality Content: High-quality, relevant, and engaging content keeps users interested and encourages interaction. Content that addresses user needs and provides value fosters loyalty and repeat visits.
Visual Appeal: A visually appealing website with a clean, modern design enhances the user experience. Consistent branding, attractive visuals, and readable typography contribute to a positive impression.
User Feedback: Incorporating user feedback mechanisms, such as surveys, reviews, and comments, allows website owners to understand user preferences and make improvements accordingly.
Focusing on user satisfaction and engagement is essential for achieving SEO success in today's digital landscape. By prioritizing intuitive navigation, responsive design, fast page load speeds, high-quality content, visual appeal, and user feedback, websites can create a positive user experience that not only improves search rankings but also fosters long-term user loyalty and satisfaction. As search engines continue to evolve, the emphasis on UX will only grow, making it a fundamental aspect of effective SEO strategies.
Voice Search Optimization: Rising importance of optimizing for voice search.
The rise of voice-activated assistants like Siri, Alexa, and Google Assistant has revolutionized the way people search for information online. Voice search is becoming increasingly popular due to its convenience and speed, prompting a significant shift in SEO strategies to accommodate this growing trend.
The Nature of Voice Queries
Voice searches tend to be more conversational and natural compared to text-based searches. Users often ask complete questions or use longer phrases, reflecting how they speak in everyday conversation. For example, instead of typing "weather Tokyo," a user might ask, "What’s the weather like in Tokyo today?" This shift necessitates a different approach to keyword research and content creation.
Importance of Natural Language Processing (NLP)
To effectively optimize for voice search, it's crucial to understand and implement natural language processing (NLP). NLP helps search engines comprehend the nuances of spoken language, including synonyms, context, and user intent. By incorporating NLP principles, websites can better align their content with the way users naturally ask questions.
Long-Tail Keywords and Questions
Given the conversational nature of voice search, focusing on long-tail keywords and question-based phrases is essential. These keywords are more specific and often reflect the exact queries users are likely to voice. Creating content that answers common questions in a natural, informative manner improves the chances of appearing in voice search results.
Structured Data and Featured Snippets
Optimizing for structured data and aiming for featured snippets (also known as position zero) can enhance visibility in voice search results. Search engines often pull answers from featured snippets to respond to voice queries. By using structured data markup, websites can help search engines understand and categorize their content more effectively.
Mobile and Local SEO
Voice searches are frequently performed on mobile devices and often include local intent, such as finding nearby businesses or services. Ensuring a mobile-friendly website and optimizing for local SEO, including updating Google My Business listings, can significantly impact voice search rankings.
Voice search optimization is becoming increasingly important as more users turn to voice-activated assistants for quick and convenient information retrieval. By focusing on natural language processing, long-tail keywords, structured data, and local SEO, businesses can enhance their visibility and performance in voice search results. This shift not only improves user experience but also positions websites to thrive in the evolving landscape of search technology.
Advanced Techniques and Tools
AI-Based SEO Tools: Overview of tools that leverage AI for SEO.
AI-based SEO tools have revolutionized the way search engine optimization is performed, offering advanced capabilities that enhance efficiency and effectiveness. These tools leverage artificial intelligence, machine learning, and natural language processing to provide insights and automate tasks that were once time-consuming and complex. Here are some of the top AI-based SEO tools:
1. ChatGPT
ChatGPT is an AI-powered language model that simulates human-like interactions. It can automate content creation, generate title tags and meta descriptions, and create detailed content outlines based on search queries. Its versatility makes it a valuable tool for both beginners and advanced SEO professionals.
2. Semrush
Semrush offers a suite of AI-powered tools, including its SEO Writing Assistant, which analyzes content for SEO potential and provides real-time feedback on readability, tone, and structure. Semrush's Copilot feature helps optimize content by suggesting improvements and identifying areas for enhancement2.
3. MarketMuse
MarketMuse is a content strategy tool that uses AI to analyze search intent and generate content recommendations. It helps marketers create comprehensive content that aligns with user queries and search engine requirements, ensuring higher rankings and better engagement.
4. Clearscope
Clearscope uses natural language processing to uncover search intent and provide data-driven recommendations for on-page SEO. It analyzes competitors' content to identify common themes and questions, helping writers optimize their content for better search visibility2.
5. Frase
Frase is a content optimization tool that assists in creating SEO-friendly content by analyzing keyword usage and suggesting improvements. It helps streamline the content creation process, ensuring that the content is both engaging and optimized for search engines.
6. Shopify Magic
Shopify Magic is an AI tool designed for ecommerce SEO, helping businesses optimize their product descriptions and other content to improve search rankings and drive traffic to their online stores.
7. Yext
Yext focuses on voice search optimization, helping businesses ensure their information is accurate and up-to-date across various platforms. This is crucial for appearing in voice search results and attracting local customers.
AI-based SEO tools have transformed the SEO landscape by automating tasks, providing valuable insights, and enhancing content quality. By leveraging these tools, SEO professionals can optimize their strategies more effectively, resulting in improved search rankings and better user engagement. As AI technology continues to advance, its impact on SEO will only grow, making these tools indispensable for modern SEO practices.
Data Analytics: Using AI for better data analysis and insights.
The advent of artificial intelligence (AI) has revolutionized data analytics, enabling businesses to extract deeper insights and make more informed decisions. AI-driven data analytics leverages machine learning algorithms, natural language processing, and predictive analytics to analyze vast amounts of data efficiently and accurately.
Machine Learning Algorithms
Machine learning algorithms are at the heart of AI-powered data analytics. These algorithms can process large datasets, identify patterns, and uncover relationships that might not be immediately apparent. By continuously learning from data, these models improve their predictions and insights over time, offering a more nuanced understanding of complex datasets.
Natural Language Processing (NLP)
NLP enhances data analytics by allowing systems to interpret and analyze human language. This capability is particularly valuable for processing unstructured data, such as text from social media, customer reviews, and emails. By converting unstructured data into structured formats, NLP enables businesses to gain insights from qualitative data that were previously difficult to analyze.
Predictive Analytics
AI-driven predictive analytics uses historical data to forecast future trends and outcomes. By applying machine learning models to past data, businesses can anticipate market changes, customer behavior, and potential risks. This proactive approach helps organizations make strategic decisions, optimize operations, and improve customer satisfaction.
Automated Data Analysis
AI automates many aspects of data analysis, reducing the time and effort required for manual processing. Automated systems can quickly generate reports, visualize data trends, and provide real-time insights. This automation not only increases efficiency but also allows analysts to focus on higher-level strategic tasks.
Enhancing Decision-Making
By providing accurate and timely insights, AI-driven data analytics enhances decision-making processes. Businesses can make data-driven decisions with greater confidence, identify opportunities for growth, and address issues before they become critical. The ability to analyze data in real-time also enables more agile and responsive business strategies.
AI-powered data analytics transforms raw data into actionable insights, enabling businesses to understand complex patterns, predict future trends, and make informed decisions. By leveraging machine learning, NLP, and predictive analytics, organizations can harness the full potential of their data, driving innovation and achieving a competitive edge in the market. This evolution in data analytics represents a significant leap forward in how businesses leverage information to achieve their goals.
Automation in SEO: How AI automates repetitive SEO tasks.
Artificial intelligence (AI) has transformed the field of search engine optimization (SEO) by automating many of the time-consuming and repetitive tasks that were once manually executed. This automation not only increases efficiency but also allows SEO professionals to focus on strategic, high-impact activities. Here’s how AI is automating key SEO tasks:
Keyword Research
AI-powered tools can quickly analyze vast amounts of search data to identify relevant keywords and search terms. These tools use algorithms to discover patterns and trends in search queries, providing insights into which keywords are most effective for driving traffic. This automation streamlines the keyword research process, making it faster and more accurate.
Content Optimization
AI tools like content analyzers and optimization assistants help in creating and refining content to improve its SEO value. They can suggest keyword placements, analyze readability, and ensure content meets SEO best practices. This automation helps maintain a consistent quality of content, enhancing its relevance and searchability.
Technical SEO Audits
Conducting a technical SEO audit involves checking various aspects of a website, such as site speed, mobile-friendliness, and indexing issues. AI-driven tools can automate these audits, scanning websites to identify technical problems and provide recommendations for fixes. This ensures that websites remain optimized for search engines without requiring extensive manual effort.
Link Building
AI can assist in link-building efforts by identifying potential link opportunities and managing outreach campaigns. Automated tools can analyze backlink profiles, find high-quality link prospects, and even send personalized outreach emails. This streamlines the link-building process, making it more efficient and scalable.
Rank Tracking
Monitoring search engine rankings is a crucial but repetitive task. AI-powered rank tracking tools can automatically track keyword rankings, analyze performance trends, and generate detailed reports. This automation ensures that SEO professionals have up-to-date information on their ranking positions, enabling them to make informed decisions.
Competitor Analysis
AI tools can analyze competitors’ SEO strategies, including their keyword usage, backlink profiles, and content performance. This automation provides valuable insights into what is working for competitors and helps SEO professionals adjust their strategies accordingly.
Reporting and Analytics
Generating SEO reports is often a tedious task. AI can automate the collection and analysis of data, producing comprehensive reports that highlight key metrics and performance trends. This saves time and ensures that stakeholders receive timely and accurate information.
AI-driven automation in SEO enhances efficiency, accuracy, and scalability by taking over repetitive tasks. By leveraging AI tools for keyword research, content optimization, technical audits, link building, rank tracking, competitor analysis, and reporting, SEO professionals can focus on strategic initiatives that drive greater results. This automation not only improves productivity but also ensures a more effective and data-driven approach to SEO.
Case Studies
Success Stories: Examples of businesses that have successfully adapted to AI-powered search engines.
In today's digital landscape, businesses that have embraced AI-powered search engines have seen remarkable success. These companies have leveraged advanced AI technologies to enhance their search capabilities, improve user experience, and drive significant growth. Here are a few notable examples:
1. Google Gemini
Formerly known as Bard, Google Gemini is a standout in the world of AI search engines. By integrating AI into its search engine, Google has significantly improved the relevance and accuracy of search results1. Gemini uses natural language processing (NLP) and machine learning to understand the context and intent behind user queries, providing highly personalized and precise results. This has not only enhanced user satisfaction but also solidified Google's position as a leader in the search engine market.
2. Microsoft Copilot
Microsoft has integrated generative AI into its search engine, Copilot (formerly Bing AI). Copilot leverages AI to deliver more relevant and context-aware search results1. By analyzing user behavior and preferences, Copilot provides personalized recommendations and answers to complex queries. This has improved user engagement and retention, making Microsoft a formidable competitor in the AI-powered search engine space.
3. You.com
You.com takes a different approach by prioritizing user privacy and offering an ad-free experience. This AI-powered search engine uses advanced language models to deliver highly relevant search results while ensuring user data remains private1. By focusing on user trust and transparency, You.com has gained a loyal user base and positioned itself as a privacy-centric alternative to traditional search engines.
4. Perplexity AI
Perplexity AI excels in delivering relevant results for complex queries, including AI job search tools, AI product search, and AI for literature search. The search engine uses OpenAI's ChatGPT-3.5 for basic access and upgrades to more refined AI models for premium users1. Perplexity's personalized search features and document analysis capabilities have made it a valuable tool for users seeking detailed insights and essential information.
5. Brave Search
Brave Search is another example of a business that has successfully adapted to AI-powered search engines. By focusing on privacy and user control, Brave Search has differentiated itself from traditional search engines1. The search engine uses AI to provide relevant and accurate results while ensuring user data remains secure and private. This approach has attracted privacy-conscious users and positioned Brave Search as a strong contender in the AI-powered search engine market.
These success stories highlight the transformative power of AI in the search engine industry. By leveraging advanced AI technologies, businesses can enhance search capabilities, improve user experience, and drive growth. As AI continues to evolve, we can expect to see even more innovative solutions and success stories in the future.
Challenges and Failures: Learning from the mistakes of those who struggled with the transition.
Adopting AI-powered search engines has offered many benefits, but it hasn't been without challenges. Some businesses have faced significant obstacles during the transition, leading to notable failures. Understanding these challenges provides valuable lessons for those navigating this evolving landscape.
Resistance to Change
One major challenge has been the resistance to change. Many businesses, accustomed to traditional SEO practices, found it difficult to adapt to the AI-driven paradigm shift. This resistance often resulted in outdated strategies that failed to leverage AI's full potential. The lesson here is the importance of embracing change and being open to new technologies and methodologies.
Lack of Expertise
Another common issue was the lack of expertise in AI technologies. Implementing AI-powered SEO tools requires a deep understanding of machine learning, natural language processing, and data analytics. Companies without the necessary skills and knowledge struggled to integrate these technologies effectively. Investing in training and hiring skilled professionals is crucial for a successful transition.
Over-Reliance on Automation
While AI-driven tools can automate many SEO tasks, over-reliance on automation can be detrimental. Some businesses neglected the human element of SEO, such as creating high-quality content and engaging with users. Automation should complement, not replace, human expertise and creativity. The key is to strike a balance between AI-driven automation and human input.
Poor Data Quality
AI algorithms rely heavily on data quality. Inaccurate, incomplete, or biased data can lead to poor outcomes. Businesses that did not prioritize data quality encountered issues with their AI implementations, resulting in unreliable insights and subpar performance. Ensuring high-quality, clean data is essential for effective AI integration.
Inadequate Planning and Strategy
Some companies rushed into adopting AI technologies without adequate planning and strategy. This led to fragmented efforts and inconsistent results. A clear, well-thought-out strategy that aligns with business goals is vital for successful AI adoption. Taking the time to plan and set realistic objectives can mitigate risks and enhance outcomes.
The transition to AI-powered search engines presents numerous challenges, but learning from the mistakes of those who struggled can guide businesses toward successful adoption. Embracing change, investing in expertise, balancing automation with human input, ensuring data quality, and having a clear strategy are key lessons for navigating this technological evolution. By addressing these challenges, businesses can unlock the full potential of AI in SEO and achieve sustained growth and success.
Future Trends
Continuous Learning: How AI continues to evolve and what it means for SEO.
Artificial intelligence (AI) is characterized by its ability to learn and adapt continuously. In the realm of search engine optimization (SEO), this continuous learning has profound implications. AI's evolving nature ensures that search engines become more sophisticated, accurate, and user-centric over time.
Ongoing Algorithm Improvements
AI-driven search engines are built on machine learning algorithms that constantly refine themselves based on new data. As these algorithms process more search queries and user interactions, they learn to deliver increasingly relevant and accurate results. This ongoing improvement means that SEO practices must also evolve to keep pace with the changing algorithms.
Enhanced Understanding of User Intent
With each interaction, AI systems become better at understanding user intent. This involves recognizing the context, nuances, and specific needs behind search queries. For SEO, this evolution emphasizes the importance of creating content that genuinely addresses user needs and provides comprehensive, valuable information. Content that aligns with user intent is more likely to rank higher in search results.
Adaptation to New Search Behaviors
AI's ability to learn from emerging trends and behaviors allows it to adapt to new forms of search, such as voice and visual search. As more users turn to voice-activated assistants and image-based searches, SEO strategies must incorporate these trends. This includes optimizing for conversational queries and ensuring that content is accessible in various formats.
Personalized Search Experiences
Continuous learning enables AI to deliver personalized search experiences by analyzing user preferences and behaviors. For SEO, this means creating personalized content and experiences that cater to specific user segments. Understanding and leveraging user data can help businesses tailor their content to meet the diverse needs of their audience.
AI's continuous learning capabilities are driving significant advancements in search engine technology. For SEO, this means staying agile and adaptive, focusing on user intent, and embracing new search behaviors. By leveraging AI's evolving nature, businesses can enhance their SEO strategies, improve search rankings, and deliver more relevant and personalized user experiences. As AI continues to advance, its impact on SEO will only grow, shaping the future of digital marketing.
Predictive Analytics: Future of predictive search and its implications.
Predictive analytics, powered by artificial intelligence (AI) and machine learning, is set to revolutionize the future of search technology. By leveraging historical data and advanced algorithms, predictive search can anticipate user queries and provide rele
Tags: AI-powered Search Engines, SEO Strategies, Machine Learning in SEO, Natural Language Processing (NLP), Voice Search Optimization, Content Optimization Tools, Predictive Analytics, User Experience in SEO, Keyword Research, Search Engine Algorithms, AI and IoT Integration, AI- driven, Personalization, Augmented Reality and SEO, Virtual Assistants and SEO, SEO Automation
0 Comments