Research Techniques in Pharmacometabolomics
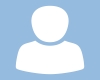
Posted by Dora West
from the Health category at
26 Mar 2024 06:57:46 am.
Pharmacometabolomics evaluates and predicts drug efficacy or toxicity by comparing the metabolic phenotype and drug-reaction phenotype of individuals before and after drug administration.
Study Process of Pharmacometabolomics
The complete pharmacometabolomics study process includes steps such as biological sample collection, biological sample preparation, data acquisition, data processing and analysis.
Biological sample selection and collection
The study objects of pharmacometabolomics can be divided into animal samples (urine, serum, plasma, tissues, organs, saliva, alveolar flush, cerebrospinal fluid, etc.) and human samples (urine, serum, plasma, saliva, tears, hair, etc.) according to their sources.
In general, liquid biological samples (urine, blood samples, saliva) are collected in milliliter quantities. Solid biological samples (tissues, organs) are collected at the milligram level. Cell culture samples are collected at the level of 1 × 106 to 1 × 107 in terms of cell number. To ensure the representativeness of the samples and to reduce the influence of individual differences in biological samples on the results, a sufficient number of samples should be collected, and attention should be paid to the matching of gender, weight, age, race, dietary habits, collection time, and sampling site between groups.
Biological sample pretreatment and preparation
The purpose of biological sample pretreatment and preparation is to extract the substance to be measured from a complex biological matrix, remove interfering impurities and transform it into a form of substance suitable for determination in order to improve sensitivity and selectivity.
Maximum retention and representation of metabolite information and reduction of interfering information (degradation, introduction of interfering substances) are the core principles of selecting biological sample preparation methods. It is necessary to select the appropriate sample extraction and preparation steps depending on the experimental purpose, the type of biological sample and the analytical instrument used for data collection.
Data acquisition
Nuclear magnetic resonance (NMR): NMR has the advantages of simple, fast and non-destructive pre-processing, but also has the defects of low sensitivity and limited dynamic range of detection.
The chromatography-mass spectrometry technique has the characteristics of high efficiency, rapidity, high sensitivity, various separation modes, and easy interpretation of results (direct correlation between variables and metabolites). However, GC/MS and LC/MS have their own drawbacks. The phenomenon of multiple peaks and multiple sources in GC/MS metabolomics studies affects the later interpretation of metabolic pathways. The main problems of LC/MS analysis are ion suppression/induction between co-flux peaks and matrix effects.
Data processing and analysis
Metabolomics data processing and analysis techniques generally include data pre-processing, pattern recognition and model evaluation, biomarker screening and identification, metabolic pathway analysis and interpretation of biological significance.
The main purpose of data pre-processing is to transform the raw spectra into a data matrix, while eliminating or minimizing errors introduced by the experimental and analytical processes and retaining most of the information relevant to the classification.
After the raw spectra are preprocessed with data, a complex multidimensional data set, i.e., a quantitative representation of metabolite profiles, will be obtained. If we want to relate it to time, pathophysiological processes and various perturbations, we need to simplify and downscale the high-dimensional complex data using multivariate statistical analysis, and summarize and summarize the sample grouping as a whole in the low-dimensional space with the help of visualized mathematical models.
Pattern recognition is one of the most commonly used multivariate analysis methods in metabolomics research, mainly including supervised classification and unsupervised classification. The essential difference between them is whether the categories to which each experimental sample belongs are known in advance. Generally speaking, supervised classification requires a large number of samples of known categories, and the commonly used methods are partial least squares discriminant analysis (PLS-DA), orthogonal partial least squares discriminant analysis (OPLS-DA), artificial neural network, SIMCA classification, etc. Unsupervised classification does not depend on the category of the samples. Unsupervised classification methods do not rely on training sets, i.e., they do not require samples with known class relationships for guidance, and look directly for point clusters or other identifiable data structures in the feature space. Principal component analysis (PCA) and cluster analysis are common unsupervised classification methods used in metabolomics research.
An important aim of metabolomics studies is to screen differential variables, i.e., potential biomarkers, from massive data and to deeply explore their biochemical significance to guide the elucidation of relevant physiopathological mechanisms. To improve the accuracy and credibility of screening differential variables, a combination of multivariate statistical analysis and univariate statistical analysis is often used as a strategy to screen and validate biomarkers using multiple metrics.
In order to find the biological meaning of these differential metabolites, the metabolic pathways involved and the metabolites, enzymes, genes, etc. associated with them need to be searched further. The metabolic pathway analysis function module of Metaboanalyst, a web-based metabolomics synthesis software, provides target metabolic pathways based on metabolic network enrichment analysis and network topology analysis based on the metabolic network score-log( p) and impact score size.
The integration of metabolomics with genomics, proteomics and other interactions is a current trend in the results of the analysis are expected to contribute to the interpretation of biological mechanisms from the perspective of biological networks and protein-gene metabolite associations, and protein-gene-metabolite associations to elucidate relevant biological mechanisms. The results will provide a basis for the interpretation of biological mechanisms from the perspective of biological networks and protein-gene metabolite associations. The integration of histological data generally requires the use of relevant databases such as HMDB, KEGG, Biocyc, Lipidmaps, PathDB, MMP, etc. The integration of histological data is usually achieved by scaffold analysis and modeling.
References
1. Kim, H. J., & Yoon, Y. R. (2014). Pharmacometabolomics: current applications and future perspectives. Translational and Clinical Pharmacology, 22(1), 8-10.
2. Neavin, D., Kaddurah-Daouk, R., & Weinshilboum, R. (2016). Pharmacometabolomics informs pharmacogenomics. Metabolomics, 12(7), 1-6.
0 Comments